eCommerce personalization is expected to converge and transform the whole industry in the coming years. By leveraging machine learning algorithms, natural language processing, and customer data analysis, AI-driven personalization techniques increase customer satisfaction, drive sales and revenue growth, enhance customer engagement, and provide a competitive edge.
The rapid evolution of consumer behavior and expectations challenges businesses aiming to attract customers who can browse hundreds of sites in mere minutes. So, AI steps in to help. They can analyze users’ data, including browsing history, purchasing behavior, click-through rates, and time spent on particular site sections. By decoding these complex patterns, AI predicts current and future buyer behavior. Its most significant value is in using this information and redefining eCommerce SEO to tailor every aspect of the company’s relationship with the customer, from the first contact to the sale.
This blog explores how AI is transforming eCommerce personalization in 2024.
What are Personalized Shopping Experiences?
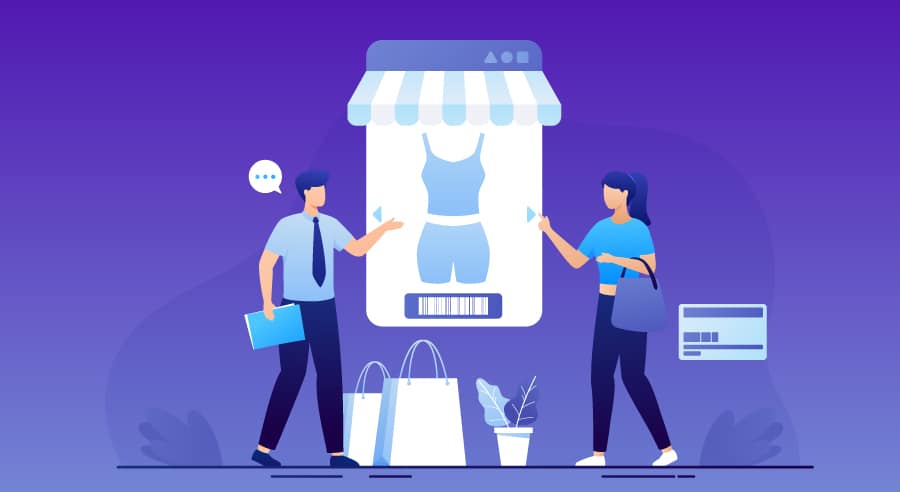
Personalized shopping experiences involve tailoring the buying process to match consumers’ preferences, buying behavior, and interests. These experiences are crafted based on data analysis of buyers’ tendencies and needs, designed to make customers feel valued. Personalization in eCommerce is vital as it involves many benefits, such as improved customer satisfaction and increased customer loyalty, thus improving sales and revenues, better customer loyalty and engagement, and giving a competitive advantage in the highly competitive market. Through personalization, eCommerce businesses can set themselves apart from their counterparts and secure consumer trust and loyalty. By applying data and analytical methods, companies can more effectively identify clients, create relevant products and services, and design memorable customer experiences that contribute to business success. It is essential for the eCommerce strategy that the core of the business model is the personalization of the purchasing process.
Advantages of Using AI in eCommerce Personalization
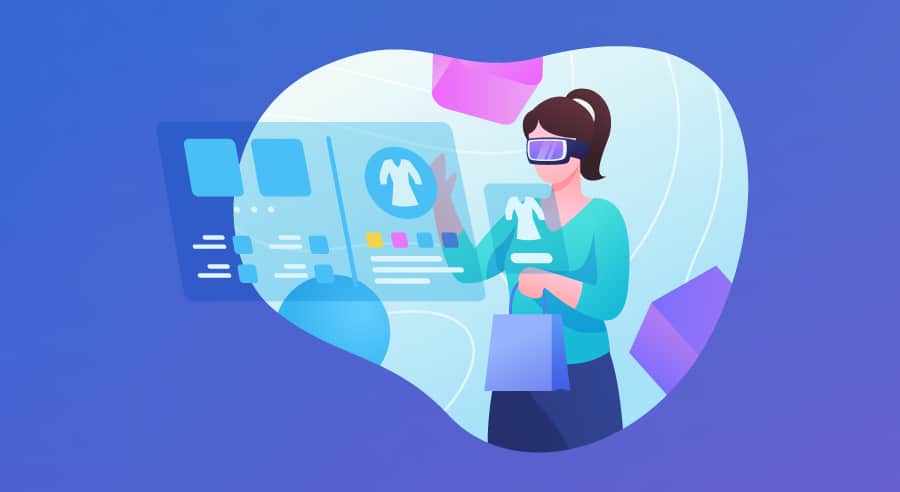
AI in eCommerce personalization offers numerous advantages, transforming how businesses engage with customers. It helps businesses deliver highly tailored shopping experiences based on individual preferences, browsing behavior, and purchase history. AI algorithms analyze vast amounts of data to predict customer needs, recommend products, and customize content, leading to increased engagement and higher conversion rates.
1. Increase Customer Satisfaction
Personalized shopping experiences meet customers’ needs and exceed their expectations by:
- Offering relevant product recommendations
- Streamlining the shopping process
- Providing tailored content and offers
- Ensuring a seamless user experience
2. Drive Sales and Revenue Growth
Increased sales and revenue growth directly result from a well-executed personalized shopping experience strategy.
- Suggesting complementary products
- Offering personalized promotions and discounts
- Enhancing the overall shopping experience
- Building trust and credibility
3. Enhance Customer Engagement
Engaged customers become brand advocates, driving word-of-mouth marketing and referrals. Personalized shopping experiences foster a deeper connection with customers, leading to loyalty and retention by:
- Understanding customer preferences and behaviors
- Offering tailored content and recommendations
- Encouraging customer feedback and interaction
- Building a community around the brand
4. Provide a Competitive Edge
A competitive edge is crucial in the crowded eCommerce landscape, and personalized shopping experiences provide a key differentiator.
- Offering unique and innovative experiences
- Demonstrating a deep understanding of customer needs
- Building strong customer relationships
- Staying ahead of industry trends and technologies
How AI Recommends Personalized Shopping Experiences
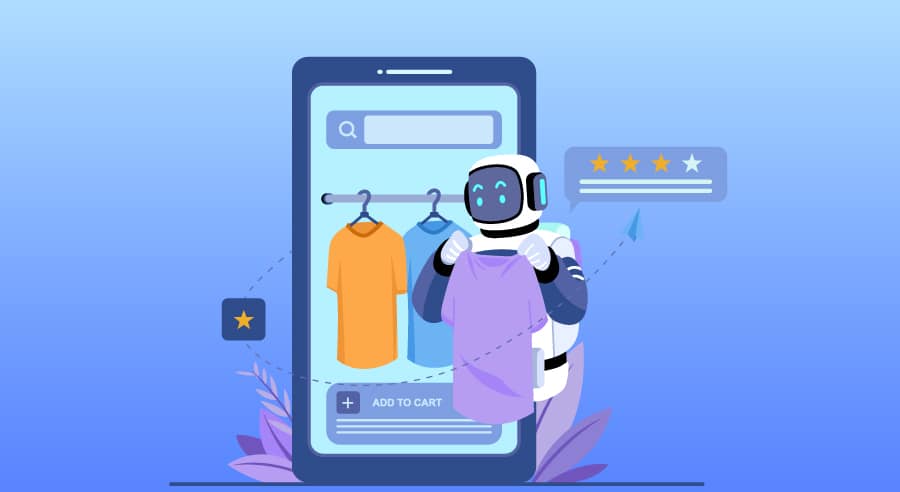
AI recommends personalized shopping experiences by analyzing customer data, such as browsing history, past purchases, and behavior patterns. Using machine learning algorithms, AI identifies trends and preferences unique to each shopper, allowing it to suggest products and services that match individual tastes and needs.
Data Analysis
Personalization is the cornerstone of modern selling techniques, and data analysis is key to achieving it. AI also analyzes customer data to determine their behavior, likes, and dislikes. It analyzes which products customers have purchased to determine which products they like. For instance, customers who shop for bicycles and running shoes are likely to also shop for cycling gloves and running shoes. Find out what customers will be typing into the search bar to understand what they are interested in or want. For instance, users typing “sustainable fashion” may seek information on environmentally friendly clothing companies. For better personalization, recommendations should be based on factors such as age, geographical location, and gender.
Machine Learning
Machine learning algorithms learn from customer data to identify patterns and preferences, enabling:
- Predict future customer actions based on past behavior. A person who has purchased a new Android smartphone will look for cases or related gadgets
- Classification of customers based on some criteria of similarity in their behaviors. For example, frequent luxury consumers will likely be interested in watches and jewelry
- Segmenting consumers with similar activities and conduct. For instance, customers who often visit the store and purchase may do so for outdoor items such as camping equipment and hiking boots
Natural Language Processing (NLP)
NLP-powered chatbots and virtual assistants use NLP to –
- Comprehending customer questions and concerns to provide accurate responses. For example, a customer might ask, “What’s the best TV for gaming?” may recommend a 4K TV with low input lag
- Suggest products based on customer interests and needs. E A customer asking about “summer fashion trends” may recommend lightweight clothing and sunglasses
Collaborative Filtering
- Recommend products with similar attributes to what you’re viewing. For instance, if you’re viewing a product with a specific feature, you may be recommended other products with the same feature
Content-Based Filtering
- Recommend products from categories you’ve previously shown interest in. For instance, if you’ve purchased from the electronics category before, you may be recommended new releases in that category
- Recommend products from brands you’ve previously purchased or shown interest in. For example, if you’ve purchased from a particular brand before, you may be recommended new products
AI-driven Personalization Techniques
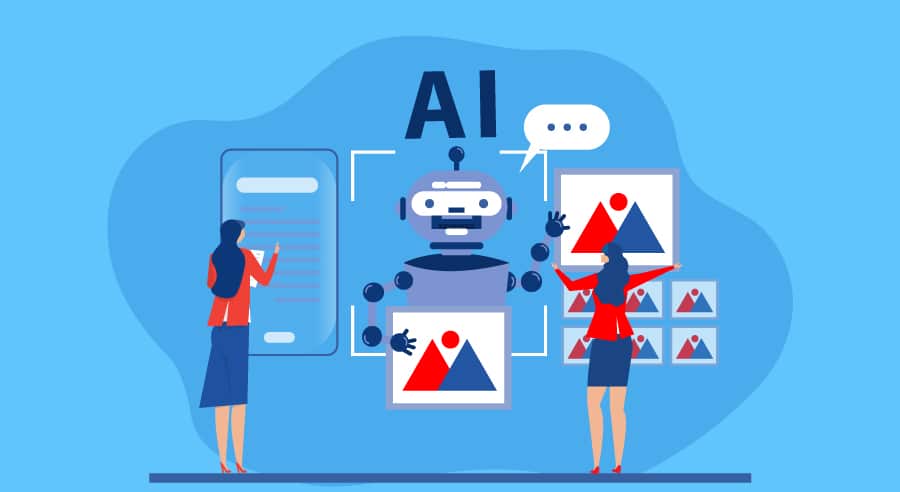
Advances in artificial intelligence lead businesses to use highly sophisticated and innovative marketing methods for their clientele. With the help of machine learning algorithms and shared customer data, it is possible to develop experiences that generate engagement, loyalty, and, consequently, increased revenues. Here are six essential techniques.
Here are six essential techniques –
AI in Product Recommendations
Recommendation engines are subprograms of an AI system that employ customer data to make product recommendations. Such algorithms learn what customers choose and what they may be interested in to guess the products they will buy. One customer, Rohan, visits an online clothing store and goes to the wear category and the men’s divider, where he sees a few shirts and jeans.
- A pair of blue shoes that go well with the shirt he added to his cart
- A few more shirts in similar styles and colors to what he browsed earlier
- A jacket that is often purchased with the jeans he views
In this example, the AI-powered recommendation engine has analyzed Rohan’s behavior and preferences to suggest relevant products, increasing his likelihood of purchasing.
Key AI technologies used
- Collaborative Filtering
- Content-Based Filtering
- Natural Language Processing (NLP)
- Deep learning
AI-Driven Targeted Promotions
Target promotions employ machine learning to learn about customer databases and deliver more tailored offers, discounts, and other enticements to clients. This approach optimizes promotional spending by targeting specific customer segments, increasing the likelihood of conversions, and maximizing long-term value.
An online retailer uses AI to analyze customer data and identify three segments –
- New customers
Offer a 10% discount on their first purchase to encourage initial sales.
- Loyal customers
Provide exclusive access to new products and loyalty rewards to retain their business.
- Inactive customers
Send personalized offers and discounts to re-engage them and prevent churn.
Key AI Technologies Used
- Segmentation
- Predictive Analytics
- Propensity Scoring
- Real-time Processing
AI-Optimized Search Results
AI-optimized search results utilize machine learning algorithms to analyze customer search queries, behavior, and preferences, ensuring that relevant products are displayed prominently and also rank in product-rich results. This streamlines product discovery enhances the shopping experience, reduces bounce rates, and increases conversions and average order value.
A customer searches for “summer dresses” on an e-commerce website. The AI-optimized search results display:
- Summer dresses from various brands have high customer ratings and reviews
- Filter options like price, brand, and size to narrow down results
- Based on the customer’s search history and preferences
- “Customers who searched for summer dresses also liked…” section
Key AI Technologies Used
- Natural Language Processing (NLP)
- Machine learning
- Knowledge Graph
- Ranking Algorithms
AI-Powered Email Personalization
AI-powered email personalization uses machine learning algorithms to analyze customer data, behavior, and preferences, enabling businesses to send tailored content, offers, and recommendations. This approach increases open, click-through, and conversion rates, fostering more robust customer relationships.
An online retailer uses AI to personalize email campaigns
- New customers receive personalized product recommendations based on their initial purchase
- Customers receive reminders with personalized offers and incentives to complete their purchases
- Valued customers receive exclusive offers, early access to new products, and loyalty rewards
- Inactive customers receive personalized offers and incentives to re-engage
Key AI technologies used
- Customer segmentation
- Content optimization
- Predictive analytics
- Real-time processing
AI-Driven Content Personalization
AI-driven content personalization uses machine learning algorithms to tailor product descriptions, images, and videos to individual customers, creating a more immersive and engaging shopping experience.
An online fashion retailer uses AI to personalize content
- AI-generated descriptions highlight features and benefits relevant to individual customers
- AI-curated visuals showcasing products in contexts relevant to individual customers
- AI-driven blog posts and videos showcasing products in scenarios aligned with individual customers’ interests
- AI-generated interactive product views allow customers to explore products from all angles
Key AI Technologies Used
- Natural Language Generation (NLG)
- Computer Vision
- Customer data analysis
- Real-time processing